How To Analyze CSV Customer Reviews With AI?
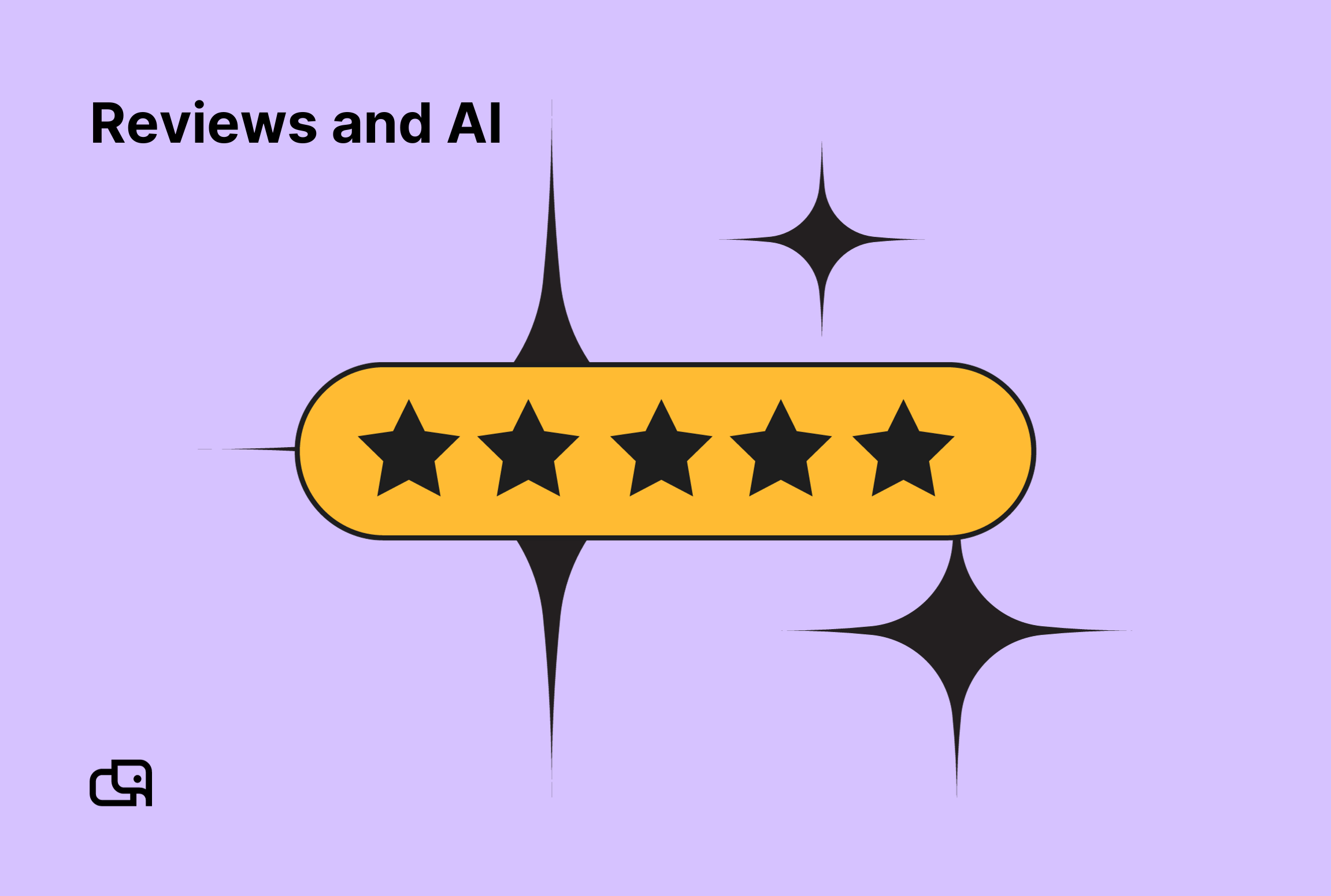
In the digital age, businesses are inundated with customer reviews in various formats, including CSV files. These reviews hold a wealth of information, but analyzing them manually can be a daunting task. Enter Artificial Intelligence (AI), a technology that can automate this process, saving time and providing valuable insights. But how exactly can you leverage AI to analyze CSV customer reviews? Let's delve into this fascinating topic.
Understanding the Importance of Analyzing Customer Reviews
Before we dive into the 'how', let's first understand the 'why'. Customer reviews are a gold mine of information. They provide direct feedback from the people who matter most to your business - your customers. Analyzing these reviews can help you understand your customers' needs, preferences, and pain points, enabling you to improve your products or services accordingly.
According to a study by Spiegel Research Center, nearly 95% of shoppers read online reviews before making a purchase. Moreover, displaying reviews can increase conversion rates by 270%. These statistics underline the importance of customer reviews in influencing purchasing decisions.
Challenges in Manual Analysis
Manually analyzing customer reviews is not only time-consuming but also prone to errors. It's nearly impossible to accurately gauge sentiments, detect patterns, and extract meaningful insights from a large volume of reviews. Furthermore, human bias can also influence the analysis.
Research conducted by the Harvard Business Review found that even when people are trained, their sentiment analysis accuracy is only about 60-65%. This is where AI comes into play, offering a more efficient and accurate solution.
How AI Can Help?
AI, with its machine learning and natural language processing capabilities, can automate the analysis of customer reviews. It can process large volumes of data quickly and accurately, identify patterns, and provide actionable insights. It can also analyze sentiments, helping you understand how your customers feel about your products or services.
According to a report by Salesforce, 51% of marketers are already using AI, and an additional 27% are even planning on incorporating this technology by 2022. This shows the growing acceptance and adoption of AI in various business operations, including customer review analysis.
Machine Learning
Machine learning, a subset of AI, involves training a model using a large amount of data. In the context of customer reviews, this could involve training the model using a CSV file containing thousands of reviews. The model learns from this data, enabling it to analyze new reviews accurately.
For instance, a machine learning model can be trained to classify reviews as positive, negative, or neutral. It can also identify key themes or topics in the reviews, such as price, quality, customer service, etc.
Natural Language Processing
Natural Language Processing (NLP) is another subset of AI that deals with the interaction between computers and human language. It enables computers to understand, interpret, and generate human language in a valuable way.
In the context of customer reviews, NLP can be used to understand the sentiment behind the reviews. For instance, it can identify whether a review is expressing happiness, frustration, disappointment, etc. This can provide deeper insights into customer sentiments, beyond just 'positive' or 'negative'.
Steps to Analyze CSV Customer Reviews With AI
Now that we have understood the 'why' and the 'how', let's look at the steps involved in analyzing CSV customer reviews with AI.
Step 1: Data Collection
The first step involves collecting customer reviews and storing them in a CSV file. This file should contain all the relevant information, such as the review text, rating, date, etc.
There are various ways to collect customer reviews. You can use web scraping tools to extract reviews from online platforms, or you can use APIs provided by review platforms. Alternatively, you can also collect reviews directly from your customers through surveys or feedback forms.
Step 2: Data Preprocessing
Once you have collected the reviews, the next step is data preprocessing. This involves cleaning the data and getting it ready for analysis. This step is crucial as the quality of your data directly impacts the accuracy of your analysis.
Data preprocessing may involve removing duplicates, handling missing values, and removing irrelevant information. It may also involve text preprocessing steps such as tokenization, stemming, and lemmatization, which are essential for NLP.
Step 3: Model Training
After preprocessing the data, the next step is to train your machine learning model. This involves feeding your preprocessed data to the model and allowing it to learn from it. The model learns to associate certain patterns in the review text with certain outcomes, such as positive or negative sentiment.
There are various machine learning algorithms that you can use for this purpose, such as logistic regression, decision trees, or neural networks. The choice of algorithm depends on your specific requirements and the nature of your data.
Step 4: Model Testing
Once your model is trained, the next step is to test it. This involves using a separate set of reviews (test set) to evaluate the performance of your model. The aim is to ensure that your model can accurately analyze new reviews that it has not seen before.
There are various metrics that you can use to evaluate your model, such as accuracy, precision, recall, and F1 score. These metrics provide a quantitative measure of your model's performance, helping you identify any areas for improvement.
Step 5: Insights Extraction
The final step involves using your trained model to analyze new customer reviews and extract insights. These insights could be in the form of sentiment scores, key themes, or any other relevant information that can help you understand your customers better.
These insights can then be used to make informed business decisions. For instance, if a large number of reviews are expressing dissatisfaction with your customer service, you may need to take steps to improve it.
Conclusion
AI offers a powerful solution for analyzing CSV customer reviews. It not only automates the process but also provides deeper and more accurate insights. By leveraging AI, businesses can gain a better understanding of their customers, improve their products or services, and ultimately, drive growth.
However, it's important to remember that AI is not a magic bullet. It's a tool that needs to be used wisely. The success of your analysis will depend on the quality of your data, the appropriateness of your machine learning algorithm, and the effectiveness of your data preprocessing steps.